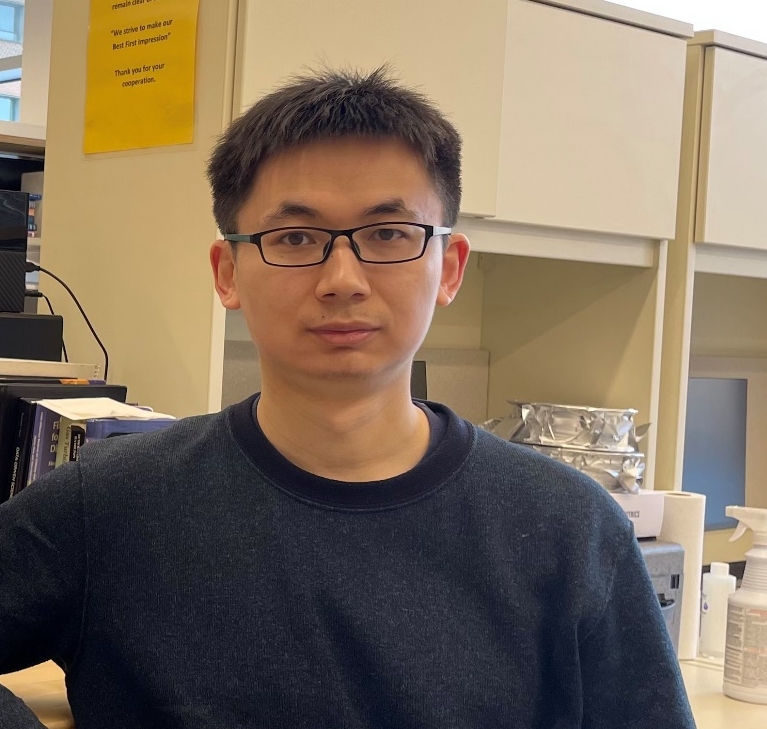
Dr. Hongyi Xu
Dr. Hongyi Xu joined University of Connecticut in 2019 as an Assistant Professor in Mechanical Engineering. His research interests include computational design of complex structure and microstructure systems, uncertainty quantification and propagation, Design for Additive Manufacturing (DfAM), stochastic microstructure characterization and reconstruction, and battery materials modeling. Dr. Xu received his PhD from Northwestern University in 2014, and worked for the Research and Advanced Engineering of Ford Motor Company from 2014 to 2019. At Ford, he led and participated in multiple research projects, including Integrated Computational Materials Engineering (ICME) of carbon fiber composites, DfAM, and vehicle body and battery safety engineering. As of March 2023, Dr. Xu serves as associate editors of three international journals. He received the NSF CAREER award, three best paper/editor’s choice awards from ASME conferences and journals, one super paper award from the tire society, and several ASME reviewer’s awards.
Computational Design of Stochastically Graded Structures for Stress Wave Manipulation
The goal of this project is to establish a computational framework for tailoring the structural stochasticity of microstructural materials to achieve desired properties. Based on this method, we propose a new structure design concept, “stochastically graded structures”, to enable effective manipulation of stress wave propagation by varying the local structural stochasticity while maintaining the material compositions and densities. The outcome of the proposed research will (i) lead to a quantitative understanding of the effect of structural stochasticity on the stress wave propagation behaviors, and (ii) accelerate the development of new structural materials for impact/blast protection.
Modeling and Design of Fluid Infilled Complex Structures for Mechanical Performances
The goal of this project is to establish a computational framework for modeling and design of components with complex inner structure and fluid infill. This project provides a novel method for generative design of fluid-structure systems to achieve unprecedented performances (e.g. ballistic/impact protection). This project includes the following tasks:
-
Featurizing and generating complex 3D structures by deep learning;
-
Developing, calibrating, and validating the fluid-structure interaction model;
-
Understanding the relationship among structural characteristics, fluid properties and filling ratio, and the nonlinear mechanical behaviors to provide guidance for design optimization of the fluid-containing structures.
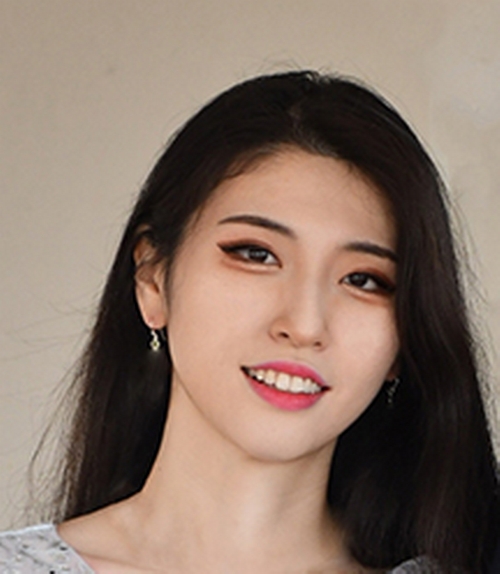
Dr. Kyungjin Kim
Kyungjin Kim joined the Department of Mechanical Engineering at the University of Connecticut in Fall 2021. Before joining, she was a postdoctoral fellow in the Lacour lab at the Swiss Federal Institute of Technology Lausanne (EPFL), working on chronic soft neuroprosthetic implants. She received her PhD from the Georgia Institute of Technology in 2018 and BS from KAIST, South Korea, in 2014, both in Mechanical Engineering. Her work focuses on novel thin film composite materials where optimizations and reliability analysis of fatigue and fracture of materials are required. She is currently developing long-term functioning soft and deformable electronic devices using vacuum-processed hermetic encapsulations and various quality control methods, ultimately to engineer the reliability of next-generation devices such as flexible and stretchable electronics, implantable bioelectronics both in the materials and device level.
Processing and Optimization of Mechanical Reliability of Thin Film Materials and Structures
Paramount importance in wearable and flexible electronics applications is especially where mechanical properties are suited to comply with dynamic motions of soft biological tissues. Yet, the majority of these applications are still under development due to a lack of reliability. We recently proposed and demonstrated a method to fabricate nanocomposite multilayer barrier films without compromising the elastic limit of mechanical deformation, consisting of thin organic and inorganic layers. These include vapor-deposited oxides, nitrides, and Parylene C barriers, solvent casting of inorganic fillers in the organic matrix, and spin-processed polyimide and fluoropolymers. We developed all our barriers to be processed at low temperatures (< 100˚C) and compatible with devices grown on thin films, transfer-printed thin devices, miniaturized silicon dies and even components from additive manufacturing. We perform an in-depth characterization of the mechanical behavior of these barrier materials under harsh environment and under mechanical loading, defining the safe design guidelines and building strategies to integrate mechanically reliable interfaces for brittle inorganic barrier materials within soft and elastic carrier substrates, including elastomers.
Manufacturing and Chronic Performance Evaluation of Flexible Thin Film Devices
We design and manufacture reliable soft interfaces for thin film applications including bioelectronic implants, fully stretchable photodiode systems, and nanocomposite-encapsulated soft actuators. Manufactured thin film devices are followed by the device performance evaluation, especially with the barrier performance encapsulating the devices, potentially offering extraordinary resilience in vivo and/or long-term biocompatibility. Our goal is to standardize the evaluation protocol with optimized processing techniques in addition to provide suitable barrier solutions to facilitate their viability. We evaluate barrier performances using various testing methods under accelerated aging environments or dynamic mechanical constraints to minimize failure issues in vitro. We recently demonstrated chronic working stretchable optoelectronic circuits combined with newly designed multilayer encapsulation that fails at the elastic limit with partial cracks yet without all-through cracking.
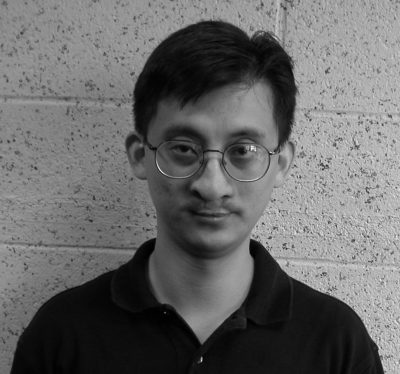
Dr. Jiong Tang
Dr. Jiong Tang is the Pratt & Whitney Professor of Advanced Materials and Processing in Department of Mechanical Engineering, University of Connecticut. He received the B.S. and M.S. degrees in Applied Mechanics from Fudan University, China, in 1989 and 1992, respectively, and the Ph.D. degree in Mechanical Engineering from the Pennsylvania State University in 2001. Prior to joining UConn in 2002, he worked in GE Research Center as a research engineer.
Dr. Tang has studied a series of research subjects including motion and vibration controls, sensing and signal processing, robotics and automation, uncertainty quantification and propagation analyses, design optimization, computational intelligence and machine learning, and modeling and analysis of multi-physics systems and processes. He has published over 200 papers in these areas. Dr. Tang’s research has been supported extensively by federal agencies and industrial companies. Dr. Tang is a fellow of the ASME.
Computational Structural Dynamics
Dr. Tang has long-term interest on computational structural dynamics. In this research, we attempt to synthesize new approach to efficiently quantify the response variation for structure with uncertainties, the outcome of which can provide vital input to robust design. For a complex structure, brute force Monte Carlo simulation for response variation analysis is infeasible since one single run may already be computationally costly. Data driven meta-modeling approaches have thus been explored to facilitate efficient emulation and statistical inference. The performance of a meta-model hinges upon both the quality and quantity of training dataset. In actual practice, however, high-fidelity data acquired from high-dimensional finite element simulation or experiment are generally scarce, which poses significant challenge to meta-model establishment. Here we take advantage of the multi-level response prediction opportunity in structural dynamic analysis, i.e., acquiring rapidly a large amount of low-fidelity data from reduced-order modeling (ROM), and acquiring accurately a small amount of high-fidelity data from full-scale finite element analysis. Specifically, we formulate a composite neural network fusion approach that can fully utilize the multi-level, heterogeneous datasets obtained. It implicitly identifies the correlation of the low- and high-fidelity datasets, which yields improved accuracy when compared with the state-of-the-art. This machine learning enabled technique can synergize multi-fidelity computational results to yield highly efficient and accurate uncertainty propagation analysis.
Vibration Control and Wave Manipulation
Dr. Tang has expertise on vibration control and wave manipulation. Wave front engineering realized through metamaterial or metasurface synthesis has attracted considerable attention in recent years. Acoustic metasurfaces have promising potentials in applications such as vibration isolation and wave guiding. Most existing devices, however, lack the tunability in real time. In this research, an adaptive acoustic metasurface taking advantage of the two-way electro-mechanical coupling of piezoelectric transducers is developed. Each surface bonded transducer (PZT) is shunted with an individually calibrated synthetic inductor to form a local resonator, which is then tuned to modify the local dispersive characteristics of each unit cell and implement discrete phase shifts. The analog synthetic inductances are integrated with digital potentiometers to realize online tunability, allowing the metasurface to be recalibrated to accommodate different incident wave frequencies or target angles of refraction without requiring any physical alteration of the host structure. Experimental validations are carried out to demonstrate successfully the bandgap performance and anomalous refraction performance of the same metasurface under different circuitry tunings.
Figure 2 Experimental investigation of adaptive metasurface for wave manipulation. Left: open-circuit; Middle: bandgap realization with metasurface; Right: anomalous wave reflection (5 degrees) realization with metasurface.
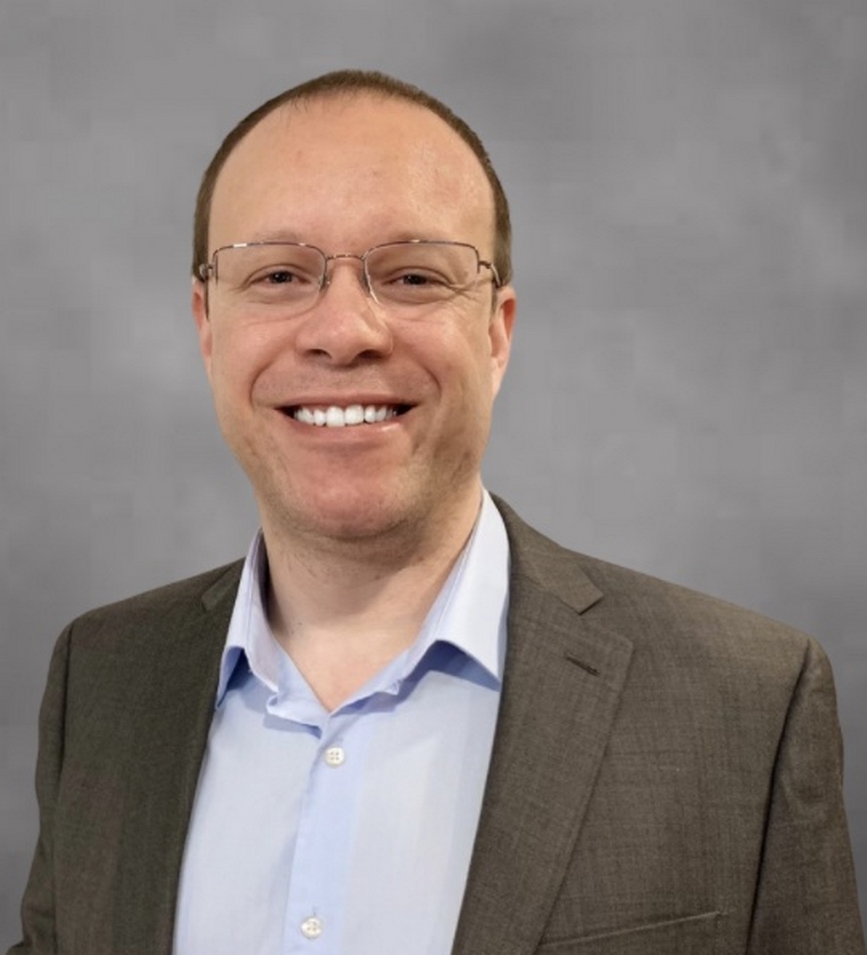
Dr. Julián Norato
Dr. Julián Norato is an Associate Professor at The University of Connecticut and a Castleman Professor in Engineering Innovation. Prior to joining UConn in 2014, he worked for Caterpillar, where he was responsible for the Product Optimization Group. He received his PhD from the University of Illinois at Urbana-Champaign. Dr. Norato is a recipient of a 2017 ONR Young Investigator Program award, a 2018 NSF CAREER Award, and the 2019 ASME Design Automation Young Investigator Award. He is a Review Editor for the Journal of Structural and Multidisciplinary Optimization and an Associate Editor for the ASME Journal of Mechanical Design. He currently serves as the Chair of the ASME Design Automation Committee.
Design of Structural Assemblies Made with Composite Materials
One focus of our work is advancing topology optimization techniques to design structural assemblies made of fiber-reinforced primitives (e.g., bars and plates). Our methods simultaneously determine the optimal layout of the primitives within a 3-dimensional design region and the fiber reinforcement of each primitive, which effectively constitutes a two-scale design. The goal of these methods is to render structural designs that are amenable to conventional composite manufacturing processes for, e.g., structures made of fiber-reinforced laminates. Our methods in this area are the first of their kind and have consistently demonstrated that the so-called black-aluminum design approach renders suboptimal designs, and that superior structures can be obtained by considering and designing the fiber reinforcement together with the structure’s topology.
Topology Optimization with regards to Stress and Fatigue Constraints
Our group has also made strides in incorporating into topology optimization local failure criteria, such as stress and fatigue-life constraints. These constraints are common and critical design requirements for high-performance engineering applications, including vehicle structures. We have formulated techniques to efficiently impose maximum stress and minimum high-cycle fatigue-life constraints for large-scale problems. Our group formulated the first density-based topology optimization technique to incorporate fatigue-life constraints in the presence of non-proportional loading, which is common in many practical applications.
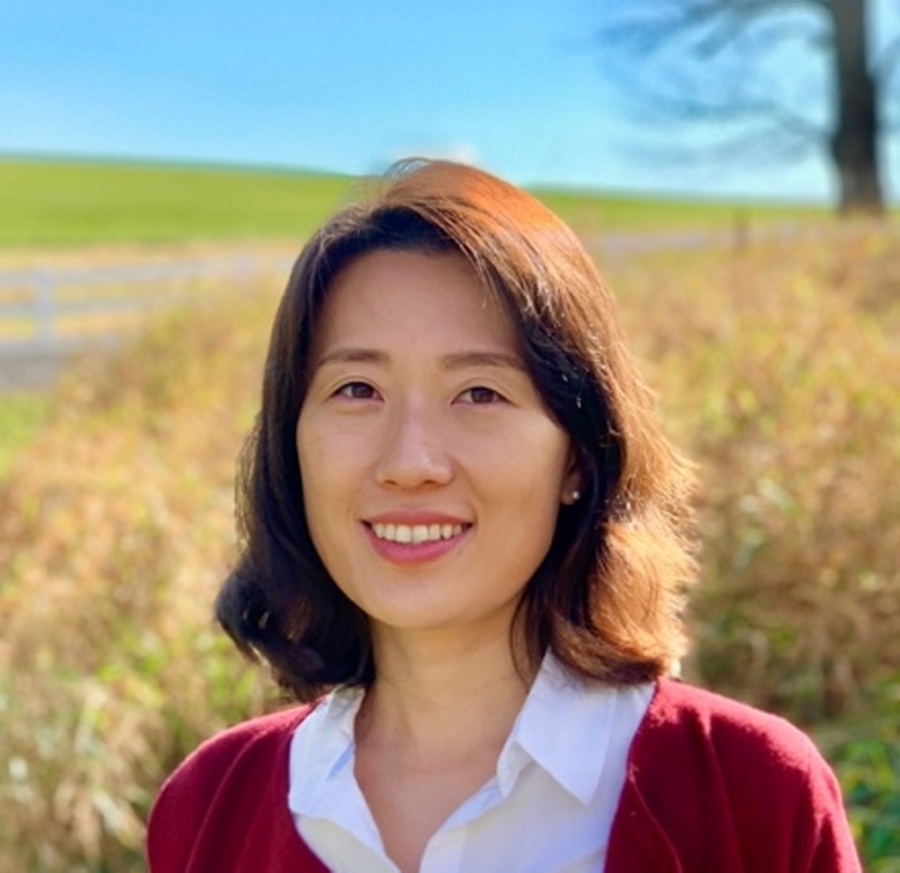
Dr. SeungYeon Kang is an assistant professor in the Mechanical Engineering department at the University of Connecticut. She obtained her B.A. degree from Cornell University in Chemical Engineering and received her Ph.D. degree in Applied Physics from Harvard University. After her graduate studies, she worked at Samsung SDI as a senior research engineer on lithium-ion batteries and at Princeton University as a postdoctoral research associate in the Mechanical and Aerospace Engineering department. Before becoming a faculty, she was the program manager for NSF’s SHAP3D additive manufacturing center at the University of Connecticut. Her current research interests include advanced laser materials processing techniques, fundamental principles and application of light-matter interaction, light-based additive manufacturing, 3D nanofabrication and energy technology.
Website: https://kang.engr.uconn.edu/
Use of Light as a Primary Printing Mechanism Enableing Relative High Speed and High-Resolution Printing
Use of light as a primary printing mechanism enables relatively high speed and high-resolution printing, when compared to other additive manufacturing (AM) techniques that typically rely on deposition of molten plastics/inks or fusion of powder-based samples. This figure demonstrates two advanced AM techniques we work on that use light to initiate various chemical reactions to print high-resolution, multimaterial 3D structures. (Top row) 100-micron resolution 3D structures printed with custom-built multi-material DLP printer. Structures are composed of polymers filled with and without carbon nanotubes. (Bottom row) Micron/sub-micron resolution 2D/3D structures printed with custom-built multi-photon SLA setup. Structures composed of silver metal structures on a 2D substrate or embedded in a polymer matrix.
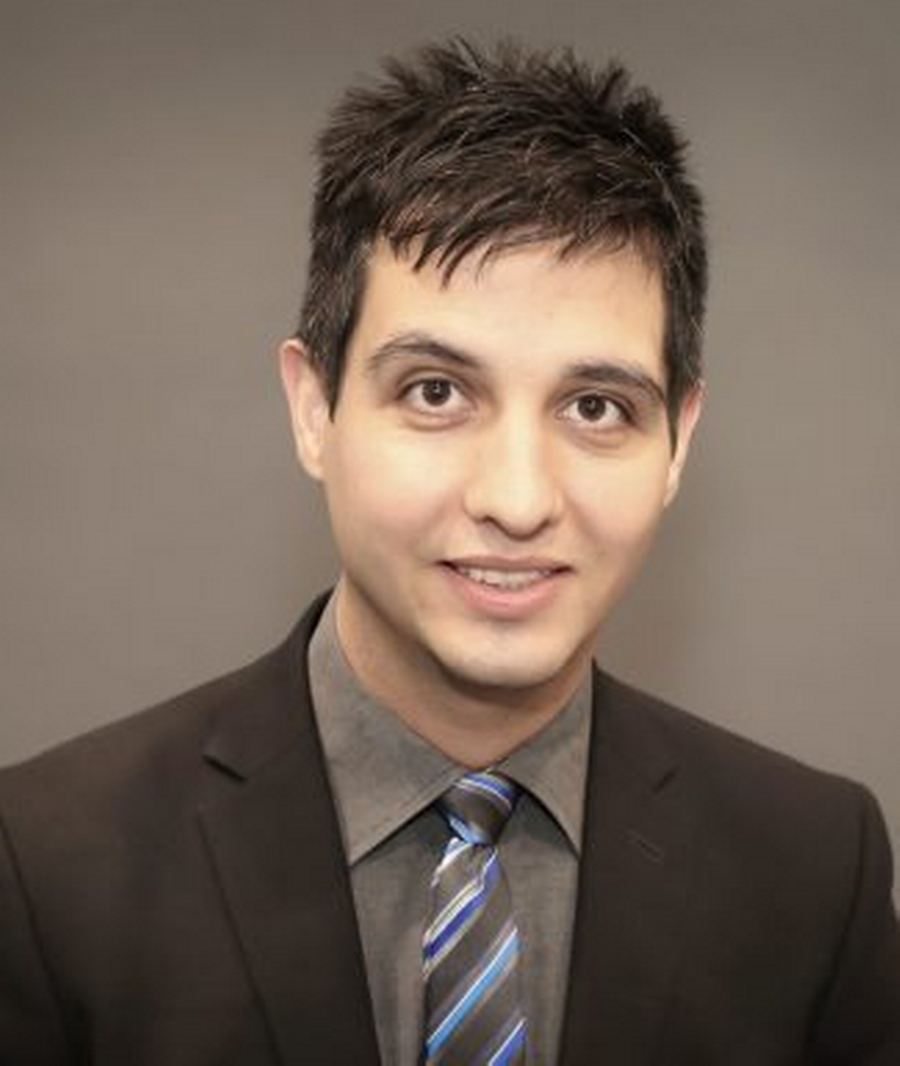
Dr. Farhad Imani
Farhad Imani is an Assistant Professor in the Department of Mechanical Engineering at the University of Connecticut (UConn). He received his Dual-title Ph.D. in Industrial Engineering and Operations Research from the Pennsylvania State University in 2020. He is an expert on sensing, control and automation, computational intelligence, and cognitive learning with applications in advanced manufacturing. His research interests encompass cyber-physical systems, privacy, and cyber security.
Website: https://imani.lab.uconn.edu/
Project 1
The ubiquitous data enabled through collaborative sensing offers an unparalleled opportunity for revamping ARMY operations. Captured sensing data are not only critical to support the current combat vehicle warfighting functions such as leader-follower truck convoys and dynamic rerouting, but also are vital for the development of the next-generation autonomous ground systems, including multi-domain launchers and advanced reconnaissance vehicles. Artificial intelligence (AI) as a key enabler leverages collaborative sensing data to learn new insights in building autonomous platforms and realize manned and unmanned teaming. However, AI algorithms, in particular supervised learning, are empowered by high-quality training data that need to be rigorously acquired to realize robust and resilient autonomous systems. In this project, we propose an intelligent human-in-the-loop system to investigate informative data acquisition by incorporating various dependencies in crowdsourced collaborative sensing.
Project 2
The capability of additive manufacturing (AM) to fabricate intricate geometry parts from hard-to-process materials manifests the potential to revolutionize production for the next generation of combat vehicles. Machine learning (ML) methods are recently invested to establish a framework that integrates sensor-based monitoring with closed-loop control to detect and subsequently correct the process drift and anomalies toward first-time-right printing. However, there are critical challenges making it difficult for ML to process data as generated, such as Transferability, Computation Efficiency and Robustness, and Reasoning and Decision Making. To achieve a cognitive system with real-time performance and robustness in AM, we redesign algorithms using strategies that more closely model the human brain. We introduce a neurally-inspired hyperdimensional computing system that mimics important brain functionalities toward high-efficiency and noise-tolerant computation.